In this post I will briefly introduce GOPHER – GenOmic Profile-model compreHensive EvaluatoR (yes, anything can be used in an abbreviation). I together with a class/labmate – Amber and my PI worked on developing this framework for analysing which training strategies to use for sequence-to-function deep learning models in epigenetics.
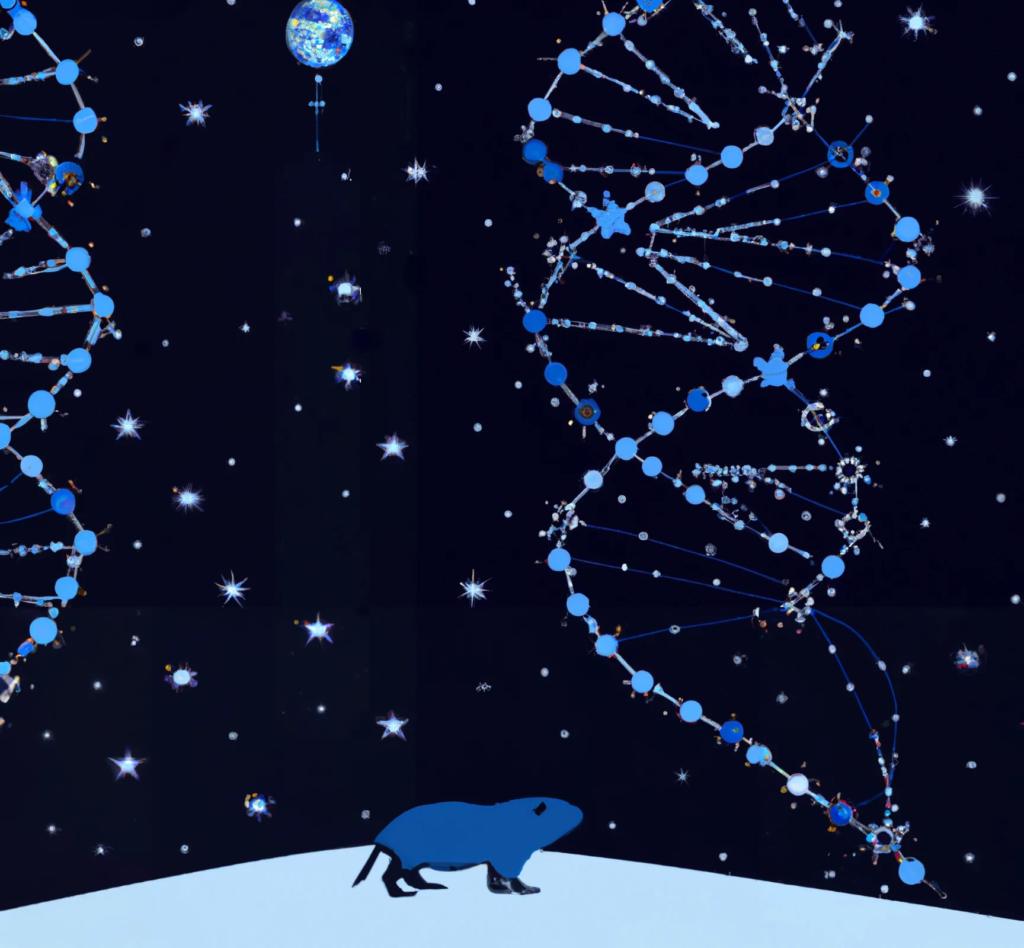
We conducted a systematic analysis of various factors and design choices involved in training such models and spent some time thinking how we can fairly evaluate them as well.
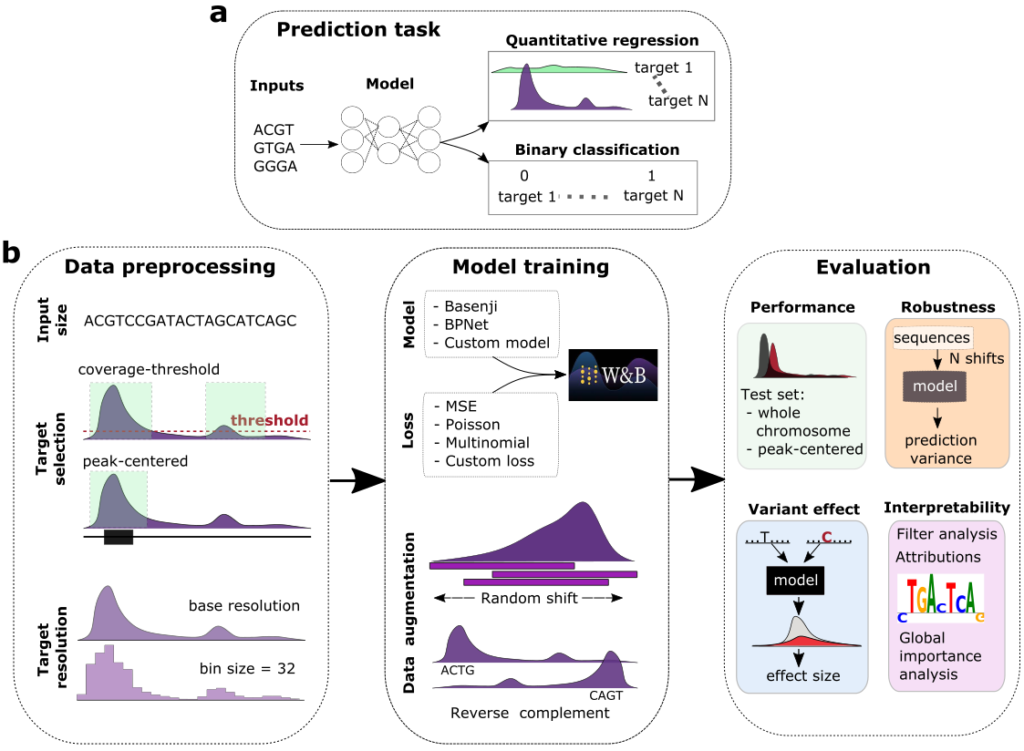
A very brief summary of the paper conclusions would be that quantitative models (predicting normalized read counts in a regression task) seem to outperform binary models (classifying each sequence to active or not active categories) in terms of performance, out-of-distribution generalization and interpretability (filter matches to JASPAR and how well they capture motif interactions).
You can read the full preprint here.